Computational analysis of microarray data
Microarray experiments are providing unprecedented quantities of genome-wide data on gene-expression patterns. Although this technique has been enthusiastically developed and applied in many biological contexts, the management and analysis of the millions of data points that result from these experiments has received less attention. Sophisticated computational tools are available, but the methods that are used to analyse the data can have a profound influence on the interpretation of the results. A basic understanding of these computational tools is therefore required for optimal experimental design and meaningful data analysis.
This is a preview of subscription content, access via your institution
Access options
Subscribe to this journal
Receive 12 print issues and online access
206,07 € per year
only 17,17 € per issue
Buy this article
- Purchase on SpringerLink
- Instant access to full article PDF
Prices may be subject to local taxes which are calculated during checkout
Similar content being viewed by others
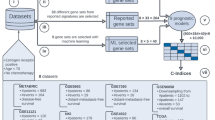
Re-evaluation of publicly available gene-expression databases using machine-learning yields a maximum prognostic power in breast cancer
Article Open access 05 October 2023
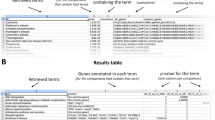
reString: an open-source Python software to perform automatic functional enrichment retrieval, results aggregation and data visualization
Article Open access 06 December 2021
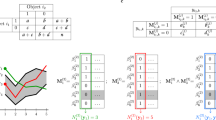
Band-based similarity indices for gene expression classification and clustering
Article Open access 03 November 2021
References
- Velculescu, V. E., Zhang, L., Vogelstein, B. & Kinzler, K. W. Serial analysis of gene expression. Science270, 484–487 (1995). ArticleCASPubMedGoogle Scholar
- Lockhart, D. J. et al. Expression monitoring by hybridization to high-density oligonucleotide arrays. Nature Biotechnol.14, 1675–1680 (1996). ArticleCASGoogle Scholar
- Schena, M., Shalon, D., Davis, R. W. & Brown, P. O. Quantitative monitoring of gene expression patterns with complementary DNA microarray. Science270, 467–470 (1995). ArticleCASPubMedGoogle Scholar
- Schena, M. et al. Parallel human genome analysis: microarray-based expression monitoring of 1000 genes. Proc. Natl Acad. Sci. USA93, 10614–10619 (1996). ArticleCASPubMedPubMed CentralGoogle Scholar
- Wen, X. et al. Large-scale temporal gene expression mapping of central nervous system development. Proc. Natl Acad. Sci. USA95, 334–339 (1998).This is one of the first analyses of large-scale gene expression — in this case, RT–PCR data — using clustering and data-mining techniques. It elegantly shows how integrating the results derived using various distance metrics can reveal different but meaningful patterns in the data.ArticleCASPubMedPubMed CentralGoogle Scholar
- Michaels, G. S. et al. Cluster analysis and data visualization of large-scale gene expression data. Pacific Symp. Biocomput.1998, 42–53 (1998). Google Scholar
- Eisen, M. B., Spellman, P. T., Brown, P. O. & Botstein, D. Cluster analysis and display of genome-wide expression patterns. Proc. Natl Acad. Sci. USA95, 14863–14868 (1998).This is an excellent demonstration of the power of hierarchical clustering to the analysis of microarray data. The authors also provide software — Cluster and Treeview — which became the standard for analysing microarray data.ArticleCASPubMedPubMed CentralGoogle Scholar
- Weinstein, J. N. et al. An information-intensive approach to the molecular pharmacology of cancer. Science275, 343–349 (1997).Weinstein and colleagues present one of the first and most elegant applications of hierarchical clustering and other data-mining and visualization techniques to the analysis of large-scale data in molecular biology.ArticleCASPubMedGoogle Scholar
- Sokal, R. R. & Michener, C. D. A statistical method for evaluating systematic relationships. Univ. Kans. Sci. Bull.38, 1409–1438 (1958). Google Scholar
- Shannon, C. C. & Weaver, W. The Mathematical Theory of Communication (Illinois Univ. Press, Illinois, 1963). Google Scholar
- Kohonen, T. Self Organizing Maps (Springer, Berlin, 1995). BookGoogle Scholar
- Tamayo, P. et al. Interpreting patterns of gene expression with self-organizing maps: methods and application to hematopoietic differentiation. Proc. Natl Acad. Sci. USA96, 2907–2912 (1999).Tamayo and colleagues use self-organizing maps (SOMs) to explore patterns of gene expression generated using Affymetrix arrays, and provide the GENECLUSTER implementation of SOMs.ArticleCASPubMedPubMed CentralGoogle Scholar
- Eisen, M. B. & Brown, P. O. DNA arrays for analysis of gene expression. Meth. Enzymol.303, 179–205 (1999). ArticleCASGoogle Scholar
- Hegde, P. et al. A concise guide to microarray analysis. Biotechniques29, 548–560 (2000). ArticleCASPubMedGoogle Scholar
- Boguski, M. S. & Schuler, G. D. ESTablishing a human transcript map. Nature Genet.10, 369–371 (1995). ArticleCASPubMedGoogle Scholar
- Quackenbush, J. et al. The TIGR gene indices: analysis of gene transcript sequences in highly sampled eukaryotic species. Nucleic Acids Res. 29, 159–164 (2001). ArticleCASPubMedPubMed CentralGoogle Scholar
- Burke, J., Wang, H., Hide, W. & Davison, D. B. Alternative gene form discovery and candidate gene selection from gene indexing projects. Genome Res.8, 276–290 (1998). ArticleCASPubMedPubMed CentralGoogle Scholar
- Ermolaeva, O. et al. Data management and analysis for gene expression arrays. Nature Genet.20, 19–23 (1998). ArticleCASPubMedGoogle Scholar
- Sherlock, G. et al. The Stanford Microarray Database. Nucleic Acids Res.29, 152–155 (2001). ArticleCASPubMedPubMed CentralGoogle Scholar
- Chen, Y., Dougherty, E. R. & Bittner, M. Ratio-based decisions and the quantitative analysis of cDNA microarray images. J. Biomed. Opt.2, 364–374 (1997). ArticleCASPubMedGoogle Scholar
- Heyer, L. J., Kruglyak, L. & Yooseph, S. Exploring expression data: identification and analysis of coexpressed genes. Genome Res.9, 1106–1115 (1999). ArticleCASPubMedPubMed CentralGoogle Scholar
- Tavazoie, S., Hughes, J. D., Campbell, M. J., Cho, R. J. & Church, G. M. Systematic determination of genetic network architecture. Nature Genet.22, 281–285 (1999). ArticleCASPubMedGoogle Scholar
- Raychaudhuri, S., Stuart, J. M. & Altman, R. B. Principal components analysis to summarize microarray experiments: application to sporulation time series. Pac. Symp. Biocomput.2000, 455–466 (2000). Google Scholar
- Brown, M. P. et al. Knowledge-based analysis of microarray gene expression data by using support vector machines. Proc. Natl Acad. Sci. USA97, 262–267 (2000).This paper shows the power of supervised techniques, in this case support vector machines, to provide additional insight into gene expression and function.ArticleCASPubMedPubMed CentralGoogle Scholar
- Golub, T. R. Molecular classification of cancer: class discovery and class prediction by gene expression monitoring. Science286, 531–537 (1999). ArticleCASPubMedGoogle Scholar
- Furey, T. S. et al. Support vector machine classification and validation of cancer tissue samples using microarray expression data. Bioinformatics16, 906–914 (2000). ArticleCASPubMedGoogle Scholar
- Hedenfalk, I. et al. Gene-expression profiles in hereditary breast cancer. N. Engl. J. Med.344, 539–548 (2001). ArticleCASPubMedGoogle Scholar
- Chatterjee, S. & Price, B. Regression Analysis by Example (John Wiley and Sons, New York, 1991). Google Scholar
- Cleveland, W. S. & Devlin, S. J. Locally weighted regression: an approach to regression analysis by local fitting. J. Am. Stat. Assoc.83, 596–610 (1988). ArticleGoogle Scholar
- Sokal, R. R. & Sneath, P. H. A. Principles of Numerical Taxonomy (W. H. Freeman & Co., San Francisco, 1963). Google Scholar
- Ward, J. H. Hierarchical grouping to optimize an objective function. J. Am. Stat. Assoc.58, 236–244 (1963). ArticleGoogle Scholar
Acknowledgements
Cluster analysis was done using the The Institute for Genomic Research MeV software package developed by A. Sturn, A. I. Saeed and J.Q., which is available at http://pga.tigr.org/tools.shtml, along with the sample data set used here. The author also thanks A. Sturn, N. H. Lee, R. L. Malek and E. Snesrud for valuable discussions and comments. This work is supported by grants from the US National Science Foundation, the US National Cancer Institute, and the US National Heart, Lung, and Blood Institute.
Author information
Authors and Affiliations
- The Institute for Genomic Research, 9,712 Medical Center Drive, Rockville, 20850, Maryland, USA John Quackenbush
- John Quackenbush